Data is at the core of every business decision today. But here’s the thing—if your data isn’t accurate, complete, or up to date, even the best analytics tools won’t help. In fact, they might lead you to the wrong conclusions. That’s why data quality in data analytics matters. High-quality data gives you insights you can trust, helping you make smarter, faster decisions.
Think about it—how many times have reporting errors caused confusion or delayed a critical project? For industries like finance, healthcare, and retail, those mistakes aren’t just frustrating—they’re costly. Poor data quality can result in compliance issues, unhappy customers, and lost revenue. On the flip side, when your data is clean and consistent, you can streamline operations, improve customer experiences, and stay ahead of competitors.
The biggest challenge? Many organizations deal with scattered data sources, manual entry mistakes, and outdated information. Fixing these issues isn’t just about technology—it’s about having the right strategy to ensure your data is accurate, consistent, and timely.
If your team relies on data to guide decisions, now’s the time to ask: How confident are you in the quality of your data? The answer can make all the difference.
Now that we’ve addressed why data matters, let’s break down what “data quality” truly means—and why you should care.
What is Data Quality?
Data quality is all about how accurate, consistent, and reliable your data is. In data analytics, good data quality means you can trust the insights you get. Without it, even the most sophisticated analytics tools can lead you down the wrong path.
Let’s break it down with a real-world example. Imagine a manufacturing company that tracks machine performance to avoid costly downtime. If the data says the machines are running smoothly when they’re not, the company might skip necessary maintenance. That oversight can lead to unexpected breakdowns, delayed orders, and higher expenses.
On the flip side, when data quality in data analytics is a priority, the company gets accurate information, plans timely maintenance, and keeps production running without hiccups.
In the end, data quality in data analytics isn’t about collecting more data—it’s about having the right data. With accurate and up-to-date information, you can make decisions that truly support your business goals and avoid costly missteps.
Also Read: Understanding Production Analysis
Understanding data quality is one thing—seeing how it drives business success is where the real value begins to show.
Data Quality’s Role in Analytics Success
Good data is more than just numbers—it’s the foundation of smart decisions and smooth operations. When your data is accurate and reliable, your analytics deliver insights you can act on with confidence. Here’s how data quality in data analytics directly impacts business success across key areas:
Business Impact
Reliable data empowers better decision-making. When you can trust the information at hand, you gain insights that help you stay ahead of competitors. For example, understanding customer preferences through accurate data allows you to tailor products and services more effectively.
Quality data also sparks innovation. Recognizing trustworthy patterns in your data can lead to new ideas and opportunities you might have otherwise missed. Plus, clean data streamlines operations—fewer errors mean your team can focus on growing the business, not fixing mistakes.
Technical Foundation
Analytics tools are only as good as the data they process. High-quality data ensures that forecasts and trends reflect what’s really happening, not skewed or outdated information. This accuracy is essential for business intelligence systems that companies rely on for critical decisions.
Data pipelines—how your data flows from one system to another—must be solid. Inconsistent or messy data disrupts these pipelines, leading to unreliable analytics. Prioritizing data quality in data analytics helps keep everything running smoothly and ensures your analytics tools deliver accurate results.
Risk Management
Mistakes in your data can be expensive. Incorrect financial reports or flawed customer information can lead to costly errors, compliance violations, and reputational damage. Ensuring data accuracy helps prevent these issues, saving both time and money.
Regulatory compliance is another big reason to focus on data quality. Industries like healthcare and finance face strict data regulations, and poor data can result in hefty fines. High-quality data also strengthens your security measures, protecting sensitive information from potential breaches.
Process Enhancement
Accurate data improves the way your business operates. Automated systems, which depend on real-time information, make faster and more accurate decisions when data quality is high. This reliability is especially important in industries where timing is everything.
Consistency across departments is just as vital. When sales, marketing, and operations all work from the same reliable data, communication improves, and everyone stays aligned. That means fewer misunderstandings and more efficient workflows.
So, what defines good data? Let’s explore the key characteristics that separate useful data from misleading information.
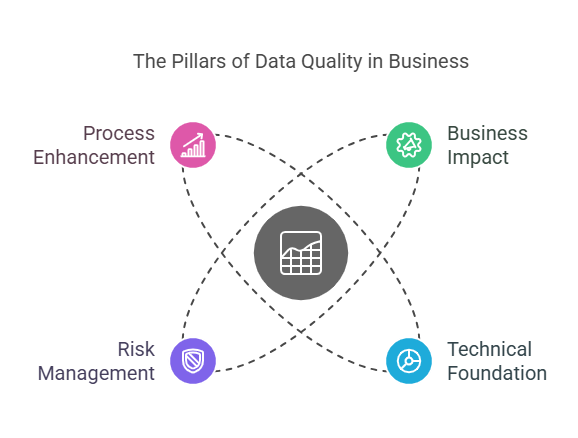
Key Characteristics and Dimensions of Data Quality
To truly benefit from data quality in data analytics, it’s important to understand its core dimensions. Each plays a vital role in ensuring data delivers meaningful insights without causing confusion or errors.
- Accuracy
Accuracy means the data correctly represents real-world situations. In other words, what the data shows should reflect what’s actually happening. For example, if a customer’s address is wrong in your system, deliveries could go to the wrong location, causing frustration and lost business. Using verified sources and double-checking entries helps maintain accuracy, ensuring your decisions are based on facts, not flawed information.
- Completeness
Completeness is about having all the necessary data available. Missing information can lead to incomplete analyses and poor decisions. Imagine a sales report missing customer contact details—your marketing team won’t be able to follow up effectively. Ensuring mandatory fields are filled prevents these gaps, giving you a full picture to work with.
- Consistency
Data should be the same across all systems and platforms. Inconsistencies can cause confusion and lead to mistakes. For instance, if a customer’s payment status shows “paid” in one system but “pending” in another, it can create billing errors. By keeping data uniform across sources, you ensure everyone in the organization works with the same information.
- Timeliness
Data loses value if it’s outdated. Timeliness means having current information available when you need it. For example, stock level data must be updated in real-time to prevent overselling. Prioritizing timely data ensures you’re making decisions based on what’s happening now—not last week or last month.
- Uniqueness
Duplicate data can skew analytics and waste resources. Uniqueness ensures each data entry is recorded only once. In a customer database, duplicates might result in sending the same promotion to someone multiple times, which can be both annoying for the customer and costly for the business. Cleaning data to remove redundancies keeps records clear and accurate.
- Validity
Data must follow defined formats and meet specific business rules. For example, a phone number should contain the correct number of digits, and a date field should include valid dates. Invalid data not only disrupts processes but also affects the quality of insights. Setting validation rules during data entry helps prevent these issues.
Also Read: What is Cloud Data Analytics?
Knowing the traits of quality data is helpful—but how do you measure it? Here’s how to assess your data’s reliability.
.png)
How to Determine Data Quality?
Knowing whether your data is trustworthy is essential for making informed decisions. Without regular checks, it’s easy for errors to slip in, leading to poor insights and costly mistakes. Here’s how you can effectively assess data quality in data analytics and keep your information reliable.
Use Data Quality Metrics and Assessments
Measuring data quality starts with tracking key metrics. These include accuracy rates, the percentage of missing data, and how often errors occur. For example, if customer addresses are often incorrect, it’s a sign your data entry process needs attention. Regular assessments, like routine data audits, help you spot these issues early and keep your data clean. By monitoring these metrics, you gain a clearer view of how dependable your data truly is.
Set Clear Standards with Data Governance
Without clear rules, data can quickly become messy. That’s where data governance comes in. It’s about creating guidelines for how data is collected, stored, and used across your organization.
For instance, you might require that every customer record includes a phone number and a valid email address. These standards help everyone follow the same process, which improves consistency and reduces errors. With strong governance, data quality in data analytics improves naturally, making your insights more reliable.
Bring in Data Quality Analysts
Sometimes, you need experts to dig deeper. Data quality analysts specialize in reviewing data for errors, inconsistencies, and gaps. They use advanced tools to spot duplicates or outdated records that automated systems might miss. Their insights not only fix current issues but also help prevent future ones. Having dedicated professionals focused on data quality ensures that you’re always working with the best information possible.
Once you know how to evaluate data, it’s time to see how improving it can benefit your business from the ground up.
Benefits of Ensuring Data Quality
Investing in data quality in data analytics pays off in many ways. High-quality data doesn’t just keep your systems running smoothly—it directly impacts business performance, customer relationships, and how efficiently you use resources. Here’s how:
.png)
- Improved Decision-Making Processes
Accurate data leads to better decisions. When you can trust the information you’re using, you avoid guesswork and base choices on facts. Whether it's forecasting sales or planning inventory, high-quality data ensures that the decisions you make are informed, timely, and effective.
- Enhanced Customer Trust and Satisfaction
Customers expect companies to know their preferences and provide accurate information. When your data is correct, you can personalize services, avoid communication mishaps, and deliver a seamless experience. Accurate billing details, timely notifications, and personalized offers build trust and keep customers coming back.
- Efficient Resource Allocation within Organizations
Good data helps you allocate resources where they’re needed most. With accurate information about sales trends or operational performance, you can focus your budget, staff, and time on the areas that will have the greatest impact. This reduces waste and improves overall efficiency.
The benefits are clear, but what common pitfalls can sabotage your data efforts? Let’s tackle those next.
Common Data Quality Issues
Even with the best intentions, data can fall short. Understanding common issues helps you prevent them from derailing your analytics and decision-making.
- Data Integration Issues
Combining data from multiple sources can be tricky. Different systems might use varying formats or definitions, making it hard to merge information accurately. For example, if one database lists “California” while another uses “CA,” inconsistencies can arise. Without proper integration, you risk incomplete or misleading insights.
- Data Entry Errors
Manual data entry is prone to mistakes—typos, missing fields, or incorrect details. These errors can lead to inaccurate reports, customer frustration, or operational delays. Imagine sending an order to the wrong address because of a simple keystroke error. Small mistakes can have big consequences.
- Lack of Standardization
When teams use different formats or naming conventions, data becomes inconsistent. One department might record dates as “MM/DD/YYYY,” while another uses “DD/MM/YYYY,” causing confusion and errors in analysis. Standardizing how data is recorded ensures everyone is on the same page.
- Data Decay
Over time, data becomes outdated. People change addresses, phone numbers, or job titles. Relying on stale information can lead to missed opportunities and inaccurate targeting. Regular updates help keep your data relevant and useful.
- Data Duplication and Redundancy
Duplicate records clog systems and distort analytics. If a customer appears twice in your database, you might waste resources contacting them multiple times or double-count sales. Removing duplicates ensures cleaner, more reliable data.
Recognizing issues is half the battle—now let’s explore proven methods to fix them and maintain quality long-term.
Link Between Data Quality and AI/ML
The effectiveness of Artificial Intelligence (AI) and Machine Learning (ML) hinges on the quality of the data used to train these models. Both technologies rely heavily on historical data to identify patterns, predict outcomes, and automate decisions. However, if the data is flawed or incomplete, the results will be unreliable, leading to poor decision-making and biased outcomes.
Why Is High-Quality Data Critical for AI/ML?
- Accurate Predictions: ML models depend on data patterns. If the data is noisy or incomplete, the algorithm might pick up the wrong patterns, resulting in inaccurate predictions.
- Training Consistency: For models to perform well, the data needs to be consistent and accurate. Any errors, gaps, or inconsistencies can cause the model to misinterpret the patterns, leading to subpar results.
Consequences of Poor Data Quality
- Inaccurate Results: AI models trained on faulty data may generate recommendations or predictions that don't align with business needs.
- Bias in Outcomes: When data is biased, the AI will continue to make biased decisions, such as favoring one group over another or producing unfair recommendations.
Ultimately, to get the most out of AI and ML, maintaining clean, accurate, and up-to-date data is essential for reliable results.
How to Measure Data Quality: KPIs and Metrics?
To evaluate whether your data is truly reliable, you need to track its quality with specific Key Performance Indicators (KPIs). These metrics help businesses assess how well their data is performing across important areas such as accuracy, completeness, and consistency.
By regularly monitoring these KPIs, you can ensure that your data supports informed decision-making.
Essential KPIs for Data Quality:
- Accuracy:
- Definition: Accuracy measures how closely data reflects real-world conditions. For instance, if customer contact details are incorrect, it can affect communication and service delivery.
- Importance: Inaccurate data can disrupt customer relationships, cause operational inefficiencies, and lead to missed business opportunities. Accurate data ensures decisions are based on reliable information.
- Completeness:
- Definition: Completeness indicates whether all necessary data points are available. For example, if customer profiles are missing contact details, the data is incomplete.
- Importance: Missing data can result in poor analysis, misleading insights, and missed opportunities. Ensuring all key fields are populated improves the overall quality of the data.
- Consistency:
- Definition: Consistency refers to uniformity in how data is recorded and represented across systems. For instance, as mentioned above, date formats (MM/DD/YYYY vs. DD/MM/YYYY) should be consistent.
- Importance: Inconsistent data can lead to confusion, errors in analysis, and problems when merging datasets.
- Timeliness:
- Definition: Timeliness refers to how current the data is. Data loses its value when it’s outdated. For example, stock levels that aren’t updated in real-time can cause inventory errors.
- Importance: Timely data enables businesses to make decisions based on the most up-to-date information, improving responsiveness and operational effectiveness.
By regularly tracking these KPIs, businesses can stay on top of their data’s quality, identify any gaps, and make informed decisions based on reliable and consistent data.
.png)
Technologies for Effective Data Quality Management
Managing data quality involves leveraging the right mix of tools, technologies, and strategies to automate data correction, monitor quality, and ensure consistency across various platforms. Without the proper tools, maintaining high-quality data becomes challenging and time-consuming.
Key Features of Data Quality Tools:
- Data Cleansing: Tools that automatically identify and correct data errors—such as typos or formatting mistakes—ensure data stays accurate and complete.
- Real-Time Monitoring: Some data quality tools offer continuous monitoring, flagging data issues as soon as they occur, helping businesses address problems before they affect operations.
- Automated Data Validation: Automated systems help businesses detect inconsistencies early, ensuring that only accurate data is used for analysis.
- Data Integration: Integration tools help unify data from multiple sources, maintaining consistency and reliability across departments and systems.
These tools are essential for businesses aiming to maintain high-quality data, streamline processes, and reduce human error in data management.
Data Quality Challenges in Cloud and Big Data Environments
Handling data quality in cloud systems and big data environments can be particularly challenging due to the sheer volume, variety, and speed of data generation. Cloud platforms often deal with data from multiple sources, which can result in fragmented or inconsistent data. In big data environments, the massive scale of data can make it difficult to maintain data integrity over time.
Key Data Quality Challenges
- Data Fragmentation: When data is sourced from multiple platforms, it may exist in different formats or be stored in silos, causing discrepancies and inconsistencies.
- Volume and Scale: The massive amounts of data generated in big data environments can overwhelm traditional management systems, making it difficult to track and maintain data quality.
Solutions for Managing Data Quality
- Data Integration: Tools that bring data from multiple sources together help maintain consistency across the organization, ensuring that all teams work from the same reliable data.
- Data Governance: Establishing data governance frameworks allows organizations to set guidelines for how data should be handled across departments, improving consistency and reliability.
- Real-Time Validation: Validating incoming data in real time ensures that data issues are addressed as soon as they arise, preventing problems from escalating.
Managing data quality in these environments requires strong tools and strategies for seamless data integration, continuous monitoring, and proactive validation.
Future Trends in Data Quality Management
The future of data quality management is being driven by the ongoing development of AI and automation technologies. As businesses continue to collect vast amounts of data, advanced tools like generative AI are expected to play a crucial role in improving data quality by automating previously manual tasks.
How Generative AI Will Enhance Data Quality:
- Automated Data Cleansing: Generative AI can automatically detect and correct errors at scale, reducing the need for manual intervention and improving efficiency.
- Predictive Data Quality: AI can analyze historical data and predict potential issues before they arise, allowing businesses to address problems proactively and maintain data integrity.
These trends demonstrate how AI and automation are poised to revolutionize data quality management, making processes faster, more accurate, and more efficient.
Best Practices for Data Quality Improvement
Improving data quality in data analytics is an ongoing process. These best practices help you maintain reliable data that drives better decisions and business outcomes.
Standardizing Data Formats and Validation Rules
Set clear guidelines for how data should be recorded and formatted. Use validation rules to catch errors during entry, such as requiring phone numbers to follow a specific format. This ensures consistency and reduces mistakes from the start.
Conducting Regular Data Quality Checks and Audits
Routine checks help identify and fix issues before they affect your analytics. Schedule audits to spot inconsistencies, outdated information, or duplicates. Proactive monitoring keeps your data clean and reliable.
Implementing Data Governance Policies
Strong data governance sets the rules for how data is collected, stored, and used. Clear policies ensure everyone follows the same standards, improving consistency and reducing errors. Assign roles and responsibilities to keep data management organized.
Implementing AI-Driven Data Cleansing Techniques
Artificial intelligence can help detect patterns and errors faster than manual checks. AI tools can identify duplicates, correct inconsistencies, and flag outdated information, making data cleansing more efficient and accurate.
Also Read: Understanding What is Data Reliability and Best Practices
While best practices help, having the right platform makes all the difference—here’s how INSIA ensures data you can rely on.
Mastering Data Quality: How INSIA's Platform Ensures Analytics You Can Trust?
Managing data from multiple systems can be overwhelming. Inconsistent information, manual processes, and data silos often lead to mistakes that slow down decisions and hurt business outcomes.
INSIA.ai simplifies data management by offering a comprehensive platform that ensures data quality in data analytics—so you can focus on making informed decisions without worrying about the accuracy of your information. Here's how INSIA’s platform strengthens data quality in data analytics:
Keeping data organized and secure is crucial. INSIA’s Governance Module helps businesses take control of their data with tools designed for clarity, consistency, and security.
- Role-Based Access Control (RBAC): Decide who sees what. INSIA lets you assign permissions so sensitive information stays in the right hands.
- Data Pipeline Management: No more chasing data across systems. INSIA ensures your data flows smoothly, securely, and efficiently.
- Business Taxonomy Setup: Define your data in terms your business understands. With customizable categories, teams speak the same data language.
- Standardized Data Definitions: Everyone uses the same definitions, reducing confusion and ensuring reports are consistent across the board.
With these features, you get a single source of truth—making decisions clearer, faster, and more accurate.
Raw data isn’t always ready for analysis. INSIA’s transformation tools clean and structure your data, so what you see is what you can trust.
- Data Cleansing Features: Automatically catch and correct errors before they affect your analytics.
- Join Functionality for Data Accuracy: Combine data from different sources without the usual headaches, ensuring information is consistent and complete.
- Automated Validation Checks: Let the system double-check your data, saving time and avoiding manual mistakes.
- Derived Columns for Consistency: Create new data points using simple tools—no coding required—so you get exactly the metrics you need.
These tools ensure your data is clean, consistent, and ready to support better decisions.
Struggling with data stuck in different platforms? INSIA’s integration capabilities bring everything together seamlessly.
- 30+ Pre-Built Connectors: Instantly connect with CRMs, ERPs, databases, and APIs—no custom coding needed.
- Single Source of Truth: Centralize your data so every team works with the same information, avoiding conflicting reports.
- Real-Time Data Validation: Stay ahead of issues with continuous checks that keep your data accurate and up to date.
- Standardized Data Formats: Say goodbye to formatting headaches—INSIA ensures consistency across all your data sources.
With all your data in one place, your analytics become faster, clearer, and more reliable.
Data security isn’t just a nice-to-have—it’s a necessity. INSIA ensures your data is safe while helping you stay compliant with industry standards.
- HIPAA, ISO/IEC 27001, and GDPR Compliance: Meet regulatory requirements with ease, no matter your industry.
- Automated Backups: Never worry about losing data—INSIA keeps your information secure and backed up regularly.
- Data Protection Measures: Built-in encryption and firewalls safeguard your sensitive information.
- Access Controls at Multiple Levels: Control access at every layer, ensuring only the right people see the right data.
With INSIA, you get peace of mind knowing your data is secure and compliant.
INSIA isn’t just a tool—it’s a solution that delivers measurable results. Here’s how businesses have improved their data quality in data analytics with INSIA:
- Alaric Enterprises: Cut manual work in half and improved inventory forecasts, ensuring timely delivery of critical pharmaceutical supplies.
- Crescent Foundry: Reduced reporting costs by 40% and doubled the speed of gaining operational insights.
- Trident Services: Achieved a 70% faster report generation, eliminating the delays caused by manual data consolidation.
Kirloskar Oil Engines: Streamlined data from various systems, reducing reporting time by 70% and enabling quicker market responses.
Conclusion
Reliable data is the foundation of smarter decisions and smoother operations. INSIA.ai helps you eliminate data headaches by centralizing information, improving accuracy, and streamlining analytics. With a single source of truth, your team can make faster decisions without second-guessing the numbers. Don’t let messy data slow you down—see how INSIA can simplify your data management and improve business outcomes.