Most manufacturers today struggle with transforming raw data into actionable insights. The disconnect between multiple data sources often results in inefficiencies, missed opportunities, and wasted resources. This fragmentation hampers decision-making and reduces your ability to respond swiftly to market changes.
The potential of big data and manufacturing working together is undeniable. The Big Data Analytics In Manufacturing Market is estimated at USD 9.07 billion in 2024 and is projected to reach USD 19.25 billion by 2029, growing at a CAGR of 16.24% during this period. This growth highlights the crucial role of big data in shaping manufacturing's future.
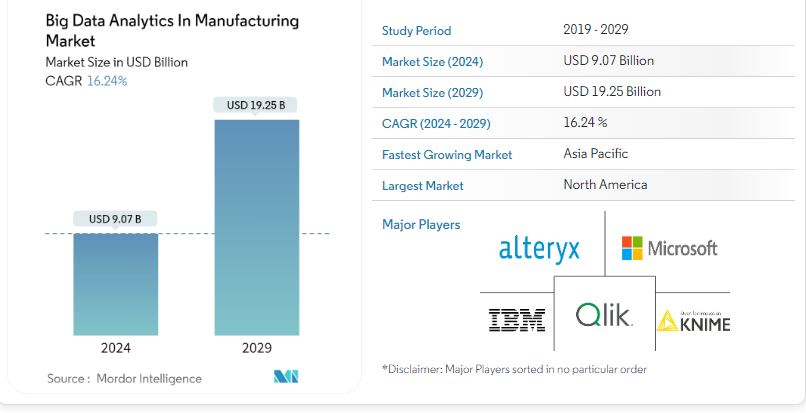
Big data analytics can identify patterns, such as a sudden increase in machine temperature or a change in vibration frequency, which could predict equipment failures before they occur. This could allow you to schedule preventive maintenance, reducing downtime and waste.
Dive into this blog to discover how big data analytics can turn challenges into opportunities, driving your manufacturing process to new heights.
Understanding Big Data in Manufacturing
Analyzing Big data and manufacturing involves vast and complex data generated throughout production. This data comes from various sources such as machinery, sensors, supply chains, quality control, and customer feedback. Integrating big data into manufacturing is a fundamental element of Industry 4.0, enabling more intelligent and efficient production processes.
Big data in the manufacturing industry is derived from numerous sources, including:
- Machinery and Equipment Sensors: Collect data on temperature, pressure, speed, and performance to monitor machine health and efficiency.
- Production Line Systems: Provide information about production rates, downtimes, and process efficiency.
- Supply Chain Management Systems: Track inventory levels, delivery schedules, and supplier performance.
- Quality Control Systems: Monitor defects, product quality, and testing results.
- Customer Feedback and Market Data: Offer insights into product performance, customer preferences, and market trends.
IoT Devices: Gather real-time data on equipment status, energy consumption, and production metrics, allowing for predictive maintenance and process optimization.
3Vs of Big Data: Volume, Velocity, and Variety
Big data holds the power to provide answers and uncover new opportunities in manufacturing. However, if you're still finding it complex, let's break it down for clarity.

Three core characteristics, often called the "3 Vs" – volume, velocity, and variety – define big data. These characteristics set big data apart from traditional data by highlighting its larger scale, faster processing speed, and diverse formats. This framework helps us understand why big data is fundamentally different and requires unique approaches for analysis and management compared to conventional data sets.
1. The First V - Volume
In manufacturing, volume refers to the enormous amount of data generated daily from various sources, including production processes, supply chain logistics, equipment sensors, and customer interactions. The scale is staggering, with industries creating up to 2.5 quintillion bytes of data every day. This immense volume emphasizes the need for advanced storage and data management systems to effectively handle and analyze the influx.
2. The Second V - Velocity
Velocity captures the speed at which data is generated and processed. Real-time data from machinery and production lines is crucial for operational efficiency in manufacturing. Equipment sensors play a significant role in providing instant feedback, allowing quick adjustments to prevent downtime. This rapid data flow demands advanced analytics tools capable of processing information in real-time, ensuring timely and informed decision-making.
For example, Ford implemented a project called Miniterms 4.0 to leverage big data, reducing unplanned downtime on its body line and saving over €1 million. This initiative, developed in collaboration with engineers from the robotics department at Universidad CEU Cardenal Herrera (CEU UCH) in Valencia, marks the first of its kind in Ford's plants.
3. The Third V - Variety
Variety addresses the different types and formats of data collected in manufacturing, from structured data like inventory records to unstructured data such as video surveillance or social media feedback. This diversity requires adaptable systems capable of handling various data types, which, when managed well, can offer comprehensive insights into operational efficiency and customer behavior.
Nestlé - one of the largest food and beverage manufacturers globally, uses big data to monitor and analyze data from its global operations, aiming to identify emerging food safety threats and business opportunities. They collect over 100 million analytical tests annually, integrating primary data with additional metadata to gain deeper insights, such as geographic and factory information.
Importance of Big Data in Manufacturing
Top manufacturers leverage big data to enhance demand forecasting and respond more accurately to market signals. According to IBM, 53% of manufacturers use big data analytics to gain a competitive edge. For example, John Deere utilized big data to save $900 million in inventory control over two years, while Coca-Cola saved $45 million annually by optimizing operations through data insights.

Let's dive deeper to learn about the importance of big data in manufacturing in detail.
1. Reduced Machine Downtime with Predictive Maintenance
Big data analytics transforms manufacturing maintenance by shifting from reactive to proactive strategies. Instead of waiting for costly equipment failures, manufacturers utilize real-time sensor data to predict potential issues, significantly reducing unexpected downtime and repair costs.
According to a Forbes study, integrating big data and the Industrial Internet of Things (IIoT) can reduce equipment breakdowns by 26% and unplanned downtime by 23%. This minimizes disruptions in production and leads to substantial cost savings. Furthermore, predictive maintenance can reduce unscheduled maintenance activities by 43%, ensuring efficient operation and accurate repair scheduling.
2. Data-Driven Enterprise Growth
Big data provides a comprehensive view of manufacturing operations, allowing comparisons across sites and identification of areas for improvement. By leveraging predictive models and simulations, manufacturers can make informed decisions about optimizing operations and expanding into new markets, ensuring growth strategies are based on real-time insights.
3. Optimizing Cost Management
Big data simplifies cost management by identifying significant overhead expenses, enabling manufacturers to implement targeted cost-saving measures. Predictive analytics enhances budget planning, ensuring resources are allocated efficiently.
4. Enhancing Customer Experience and Product Customization
Big data empowers manufacturers to align products with customer expectations by analyzing feedback and market trends. A Deloitte study indicates that a data-driven approach enhances customer satisfaction, with 36% of consumers willing to pay more for personalized products. By leveraging big data, manufacturers gain deeper insights into consumer preferences, resulting in highly customized products and services that resonate with target audiences.
Applications of Big Data in Manufacturing
Big data's real value in manufacturing lies in its ability to predict and actively solve problems. Today, it's about shifting from merely predicting outcomes to prescriptive analytics—using data to plan and prevent future disruptions.
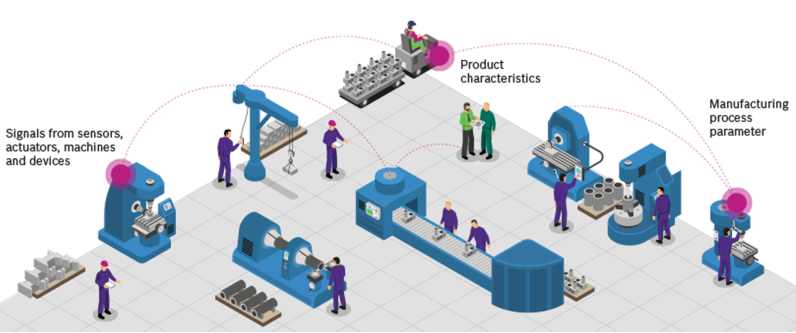
According to the same IBM report, industrial manufacturers lead the way, excelling in analytics capabilities compared to other industries, showcasing the transformative potential of big data in manufacturing.
Predictive Maintenance:
1. Price Optimization
Manufacturers can set optimal pricing by evaluating supplier costs, market demand, and customer preferences. Bosch uses big data analytics in manufacturing to enhance budget management and lower scrap costs by up to 65%.
2. Image Recognition
Big data facilitates image recognition for instant spare parts identification. A simple photo upload provides all necessary product details, boosting efficiency.
3. Quality Control
Big data enhances quality control by identifying defects early in the production process. Intel leveraged big data to improve its chip quality check process, analyzing historical data to target specific tests at the wafer level. This predictive approach significantly reduced testing time, leading to a 25% decrease in chip quality processing time. As a result, Intel achieved substantial cost savings, cutting $3 million from one core processor line, demonstrating the power of big data in optimizing manufacturing efficiency.
4. Production Forecasting
Anticipating demand shifts helps manufacturers meet market needs without overproducing or experiencing shortages. Again, Intel integrates big data and manufacturing to predict future chip demand and ensure production capacity aligns with market needs. Intel uses data-driven strategies to monitor market conditions, plan investments, and forecast semiconductor demand, ensuring they meet future production requirements efficiently.
5. Supply Chain Optimization
Big data provides insights into every stage of the supply chain, helping manufacturers respond swiftly to changes and minimize delays. Kia Motors' "Plan S" strategy integrates data from electric vehicle usage and market trends to create tailored mobility solutions.
6. Testing and Simulation
Big data supports digital twins and simulation environments, allowing manufacturers to optimize processes before full-scale production. This predictive approach ensures smoother operations and accelerates time-to-market.
Challenges in Implementing Big Data
While big data analytics has the potential to revolutionize manufacturing, it also presents several challenges. Let’s discuss some common challenges companies often face and the solutions that can help address them.

Data Volume, Variety, and Velocity (3 Vs):
The manufacturing industry generates massive data from sensors, machines, and other sources. This data exists in multiple formats—structured, semi-structured, and unstructured—making it challenging to process and analyze. Traditional data infrastructure often struggles to handle such complexity, limiting the ability to extract valuable insights effectively.
- Solution: Implement scalable cloud-based data warehouses and advanced data management tools to process diverse data formats. Utilize AI-driven algorithms to handle real-time data streams effectively.
Data Integration and Silos:
Manufacturing data is often stored in isolated systems such as SCADA (Supervisory Control and Data Acquisition) and ERP (Enterprise Resource Planning), making achieving a unified data view for analysis and decision-making challenging.
- Solution: Unify data using modern data integration platforms and middleware. Employ APIs and data lakes for seamless data flow, enabling comprehensive analysis.
Data Quality and Security:
Incomplete or incorrect data can lead to flawed insights and poor decision-making. As the volume of data grows, security concerns also increase, necessitating stronger protection measures to safeguard sensitive information and maintain data integrity.
- Solution: Implement automated data cleansing tools to ensure accuracy. Adopt robust encryption and multi-factor authentication to protect sensitive information and ensure compliance with cybersecurity standards.
Skilled Workforce:
A significant challenge in big data initiatives is finding a workforce with the necessary expertise in big data technology, data science, and analytics. This skills gap can hinder effective implementation and analysis, ultimately limiting the potential success of big data projects in manufacturing.
- Solution: Invest in training programs for existing staff and partner with educational institutions. Alternatively, collaborate with external data experts to bridge the skills gap quickly. Explore a No-code/ Low-code unified data platform for faster adoption.
Cost of Implementation:
Implementing big data analytics demands significant investment in infrastructure, software, and skilled talent, which can be a substantial initial cost, particularly for small and medium-sized manufacturers.
- Solution: Start with pilot projects to demonstrate ROI before scaling up. Consider cost-effective cloud-based big data solutions that offer scalability without significant infrastructure investment.
Future Trends in Big Data Analytics
Big Data Analytics is revolutionizing the future of the manufacturing industry. It plays a crucial role in the shift toward Industry 4.0 and lays the groundwork for Industry 5.0.
The Toyota Production System (TPS) is pivotal in modern manufacturing, focusing on efficiency and waste reduction. Its principles—like Just-in-Time production and Jidoka—enable manufacturers to respond swiftly to market demands and improve quality control.
Such analytics can be enhanced through real-time data insights, enabling predictive maintenance and optimization of supply chain processes.

As big data and manufacturing continue evolving, we can expect even more transformative applications to emerge. Let’s explore some ways Big Data Analytics is set to reshape the manufacturing landscape.
Advanced Analytics and AI-Powered Decision Making
Integrated artificial Intelligence, Big Data, and Machine Learning algorithms are revolutionizing manufacturing processes. These technologies enable advanced analytics, facilitating real-time decision-making and optimized production planning. Big Data is crucial in processing vast amounts of information, allowing for more informed and efficient operations.
Example: The BMW Group exemplifies the strategic use of advanced analytics in modern manufacturing to enhance production efficiency and maintain premium quality. Throughout the automotive manufacturing process, an immense volume of data is generated across the entire value chain. This advanced system selectively analyzes vast datasets, extracting valuable insights to refine and optimize the company's production processes. By leveraging smart data analysis, BMW has significantly improved quality control throughout its production and logistics operations.
The Rise of Digital Twins
The global digital twin market was valued at approximately USD 16.75 billion in 2023 and is expected to expand at a compound annual growth rate (CAGR) of 35.7% from 2024 to 2030. This technology is gaining momentum due to its ability to connect the physical and virtual realms effectively.

Digital twins, virtual replicas of physical machines and processes, have become increasingly sophisticated. These digital counterparts integrate sensor data and real-time performance metrics, enabling continuous simulation and optimization of production processes. Digital twins allow manufacturers to refine their operations without disrupting physical production by providing a virtual testing ground.
Closed-Loop Manufacturing and Self-Optimizing Systems
The manufacturing industry is moving towards closed-loop ecosystems, where data flows seamlessly between products, machinery, and the entire supply chain. This interconnectedness enables systems to self-optimize, making real-time adjustments based on demand fluctuations, resource availability, and product performance. The result is a more responsive and efficient manufacturing process that can autonomously adapt to changing conditions.
Example: Philips has created a closed-loop recycling system that recycles old electronics and reuses their components in new products, helping to reduce e-waste and preserve resources.
Best Practices for Big Data Implementation
Implementing Big Data in the manufacturing sector demands thorough planning and execution to achieve successful outcomes. Below are some best practices for effectively integrating Big Data into manufacturing processes:
Defining Clear Business KPIs and Measuring ROI
Setting transparent KPIs and accurately calculating ROI is crucial to validating your business's viability and profitability. By initiating a Discovery Phase, you can analyze different scenarios to understand the potential benefits Big Data can bring to your manufacturing process. This phase will equip you with all the necessary deliverables to start the implementation phase efficiently while addressing potential risks and optimizing costs.
Assessing Manufacturing Challenges
A thorough analysis of your manufacturing challenges and requirements is essential. Evaluate the quality of your final product and explore potential improvements. Consider the advantages and disadvantages of integrating Big Data into your processes and how its application can enhance your quality improvement efforts.
Implementing a Robust Big Data Engineering Process
The success of any Big Data analytics initiative relies on several critical factors:
- Hiring skilled Big Data developers to guide you through the process
- Selecting appropriate data sources
- Building a coordinated ecosystem that collects and integrates data from multiple sources
- Cleaning, aggregating, and preprocessing the manufacturing data to align with specific business objectives
- Applying Data Science, Machine Learning, or AI models
- Visualizing the extracted insights effectively
Conclusion
In summary, Big Data and manufacturing have revolutionized the industry by enhancing quality control, predicting maintenance, improving customer support, optimizing supply chains, designing better products, reducing costs, and ensuring worker safety. With the right implementation, manufacturers can make informed decisions, increase efficiency, and drive growth. As the industry advances, leveraging Big Data will be essential for maintaining competitiveness in a data-driven environment.
How to Enhance Manufacturing Efficiency with INSIA's Big Data Solutions?
INSIA helps businesses improve their manufacturing processes through Big Data analysis by offering the following solutions:
Service Engineer Utilization:
- Unified Data Platform: Handles large volumes and diverse data types efficiently.
- End-to-End Automation: Automates the entire analytics value chain, from data ingestion to insights.
- Cost Efficiency: Eliminates the need for multiple software solutions across the analytics process.
- User-Friendly Interface: No-code/low-code UI enhances user adoption and simplifies data handling.
- Accelerated Decision-Making: Provides actionable insights quickly, empowering manufacturers to make informed decisions.