Manufacturers face constant pressure to boost productivity, cut costs, and uphold quality standards—all while navigating increasingly complex operations. Traditional methods, often reliant on fragmented data, make it challenging to make timely, informed decisions. This leads to missed opportunities for optimization, inefficiencies, and rising costs.
However, the manufacturing landscape is changing rapidly. The big data market in manufacturing is projected to grow by USD 17.32 billion between 2023 and 2028, with a remarkable CAGR of 25.86%. This growth is driven by the rise of Industry 4.0 practices and the increasing use of Artificial intelligence (AI) and Machine learning (ML) technologies. These advancements allow manufacturers to optimize operations, reduce waste, and make faster, more informed decisions.
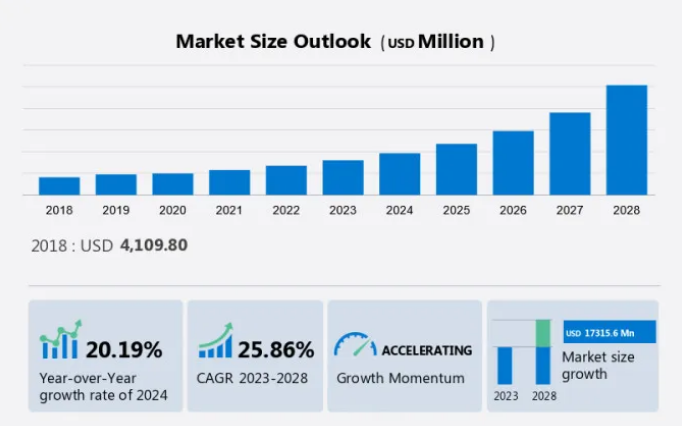
Data-driven manufacturing takes this further by integrating data from equipment, operators, and supply chains, empowering fact-based decision-making. This approach provides real-time insights, helping businesses improve performance and streamline processes.
In this article, we’ll dive into the benefits of data-driven manufacturing, explore the challenges, and offer actionable strategies to help you implement these solutions effectively. First of all, let’s discuss the basics of data-driven manufacturing.
What is Data-Driven Manufacturing
Data-driven manufacturing is more than just a buzzword; it's a transformative approach that relies on factual data and key performance indicators (KPIs) to guide decision-making. Instead of operating on guesswork, manufacturers can now use real-time data from various sources to inform their actions and improve efficiency.
Accurate data collection plays a crucial role in this process. Data comes from multiple areas, including shop floor equipment, operators, sales, services, and supply chain operations. Gathering and analyzing this data in real time allows manufacturers to pinpoint inefficiencies, track performance, and make informed adjustments that positively impact production.
This approach departs from traditional manufacturing, where decisions often rely on historical data or gut instincts. Today's manufacturers use modern tools to collect, analyze, and act on data as it happens. The difference is striking: real-time insights enable businesses to be proactive rather than reactive, which leads to optimized processes and better outcomes.
Model-Based Manufacturing vs. Data-Driven Manufacturing
When discussing advancements in manufacturing, two key approaches often come up:
- Model-based manufacturing
- Data-driven manufacturing
Both methods have their strengths but are distinct in their operations and the types of insights they provide.

Model-based manufacturing relies on simulations built from historical data to guide decisions. It's like following a blueprint, offering structure and consistency. For example, an aerospace factory might use digital models to simulate production and optimize operations. While this approach ensures repeatability, it can be less adaptable to real-time changes.
In contrast, data-driven manufacturing uses live data from machines and operators, offering flexibility. Decisions are based on current conditions, helping manufacturers quickly address issues and optimize processes in real-time.
Let’s understand better through this table.
Now that we understand data-driven manufacturing let’s explore its key benefits.
Benefits of Data-Driven Manufacturing
Embracing data-driven manufacturing gives manufacturers a competitive edge and opens doors to various operations improvements. Manufacturers can address fundamental inefficiencies and optimize processes using real-time data to guide decision-making.
Below, we’ll explore several specific benefits of adopting a data-driven approach, using examples to illustrate how each aspect can potentially improve manufacturing outcomes.
Below, we’ll explore several specific benefits of adopting a data-driven approach, using examples to illustrate how each aspect can potentially improve manufacturing outcomes.
Increased Visibility Across Operations
One of the most significant advantages of data-driven manufacturing is enhanced visibility. Manufacturers can track operations across the entire production process, from when raw materials enter the facility to when the final product leaves.
For example, Carolina Precision Manufacturing, a company making CNC Swiss-turned parts, used an IoT platform to get a clear view of its operation. With real-time data on how machines were performing and where delays were happening, they were able to make big improvements. In just one year, this led to $1.5 million in savings. The platform helped them boost production efficiency, use their machines more effectively, and improve operator accountability by providing instant updates on performance.
Minimizing Waste and Reducing Costs
Waste reduction is another critical benefit. In traditional manufacturing, inefficiencies often go unnoticed until they become costly problems. Data-driven approaches allow manufacturers to identify areas of waste much earlier.
For example, a company producing packaging materials might notice through data monitoring that a specific part of the production line uses excess material. Instead of waiting until the monthly audit to address this, the company can make real-time adjustments, reducing waste and cutting costs.
Integrating Lean Six Sigma methodologies with data analytics has effectively reduced waste. The HyDAPI (Hybrid Digitisation Approach to Process Improvement) methodology combines Six Sigma and data mining elements to enhance quality management in manufacturing processes. This approach allows manufacturers to identify non-value-adding operations and streamline processes, achieving leaner operations with less waste.
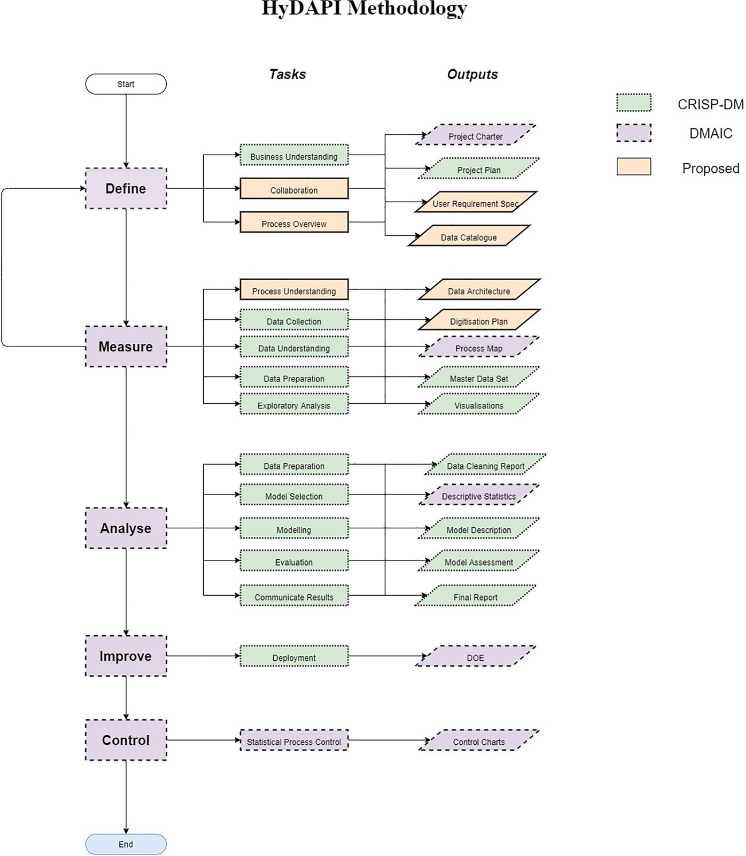
Beyond material waste, manufacturers can reduce energy consumption by monitoring machine usage and optimizing energy-intensive processes. A plant producing automotive components could use data to identify when machines are idle but still consume power. Adjusting workflows to power down machines during inactivity can save energy and reduce operational costs.
Improving Resource Utilization
Effective resource utilization is critical to achieving cost efficiency and sustainability in manufacturing. Data-driven approaches enable manufacturers to precisely monitor the use of resources such as labor, materials, and equipment.
For example, Global chemical manufacturer Celanese collaborated with Cognite and Microsoft Azure to establish a "Digital Plant of the Future." This initiative consolidated operational and engineering data into a single reliable environment, enabling better decision-making across manufacturing floors and field services. The result was improved efficiency and resource management throughout their operations.
Better Product Quality
One of the most significant shifts in data-driven manufacturing is its impact on product quality. With accurate data, manufacturers can maintain consistent quality across production runs.
For example, Boeing enhances product quality through data-driven manufacturing by leveraging data from thousands of sensors embedded in their aircraft. These sensors collect real-time information on machine performance, flight operations, and environmental factors, allowing Boeing to monitor and optimize production processes and product performance.
By analyzing this data, Boeing can identify potential issues early, make precise adjustments, and improve overall product quality. This approach enables Boeing to prevent defects, streamline maintenance, and ensure that its aircraft meet high safety and efficiency standards throughout their lifecycle.
Optimizing Risk and Ensuring Safety
Manufacturing environments are complex, and risks are ever-present, whether related to worker safety or machine malfunctions. Data-driven manufacturing helps reduce these risks by providing real-time insights into potential hazards.
For example, Mercedes-Benz uses Industry 4.0 and data-driven manufacturing to optimize risk management and ensure safety across its production processes. Through smart factories that seamlessly connect humans, machines, and workflows, the company enables real-time monitoring to identify and address anomalies instantly. Comprehensive digitalization enhances proactive safety protocols, minimizing operational risks and improving workplace security. The integration of a fully digital process chain ensures resource efficiency by tracking components with precision and preventing mismanagement.
Minimizing Downtime and Increased Machine Efficiency
Manufacturers can use data to optimize maintenance schedules, reducing the likelihood of machine breakdowns. A factory producing heavy machinery can track machine performance over time, identifying wear and tear before it leads to a costly failure. By scheduling preventive maintenance when needed rather than after a breakdown, manufacturers can ensure worker safety, minimize downtime, and avoid unexpected costs.
For example, Chevron’s plan to implement predictive maintenance solutions in its oil fields and refineries is a strong example of data-driven manufacturing. The company can gather real-time data on machine performance by equipping machinery like heat exchangers with IoT sensors. This data lets Chevron anticipate issues before they lead to breakdowns, helping them avoid costly repairs and downtime. The connection to data-driven manufacturing lies in using live data to optimize operations and improve equipment reliability and efficiency through proactive decision-making.
Reducing Overall Costs and Maximizing Profitability
A well-implemented data-driven manufacturing system can significantly reduce overall costs. While initial setup and integration may require investment, the long-term savings far outweigh the costs.

For example, Siemens uses digital twin technology, which creates virtual copies of its manufacturing processes. This allows Siemens to test new ideas without physical prototypes, saving time and money. The system also monitors production in real time, spotting problems early. This leads to better product quality and helps make the entire operation more efficient, lowering overall costs.
While the benefits are clear, implementing data-driven processes does come with challenges.
Challenges in Data-Driven Manufacturing
While data-driven manufacturing offers significant benefits, implementing it effectively has several challenges that manufacturers must address. Below are some common hurdles and how they impact the journey toward full data integration.
Siloed Data and Legacy Systems
One of the biggest obstacles in data-driven manufacturing is dealing with siloed data and outdated systems. Many manufacturers have legacy equipment and systems that are never designed to communicate with modern data platforms. For example, older machinery may not be equipped with sensors or IoT capabilities, making it difficult to gather real-time data. This data source fragmentation can limit manufacturers' visibility across their entire operation, forcing them to rely on isolated datasets that don't provide a complete picture.
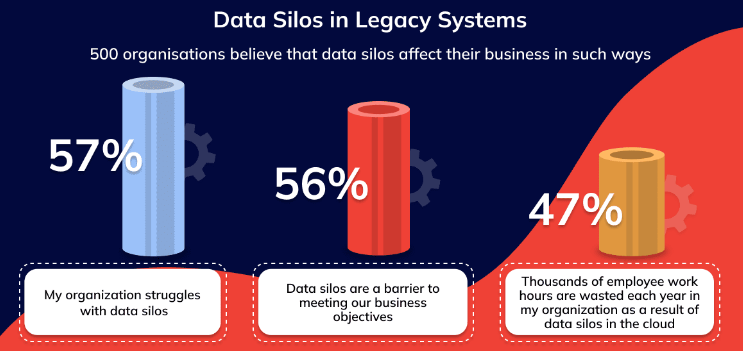
To overcome this, manufacturers often must retrofit older machines with modern sensors or adopt middleware that helps bridge the gap between legacy systems and new data platforms. Without addressing these silos, it's challenging to harness the power of data fully.
Data Quality in Data-Driven Manufacturing
In data-driven manufacturing, high-quality data is essential for making accurate decisions. However, keeping data reliable can be challenging. Data comes from various sources, like IoT devices, sensors, and older systems, which often have differences in format, accuracy, and consistency. When data is incorrect or incomplete, it can lead to mistakes and inefficiencies in production.
Manufacturers need good data management practices, such as regular data cleaning, validation, and standardization, to maintain data quality. While real-time data is valuable, it can also bring errors that, if not managed, could misguide operations or create bottlenecks. Investing in tools to improve data quality and training staff in best practices can help manufacturers get clear, actionable insights.
Without a focus on data quality, the advantages of data-driven manufacturing may be lost, as poor data can lead to costly mistakes. Reliable data is the backbone of effective analytics, allowing manufacturers to benefit fully from a data-driven approach.
Data Storage and Management
The sheer volume of data generated by modern manufacturing processes poses its challenge. With thousands of sensors collecting data every second, manufacturers must ensure they have the infrastructure to store, process, and manage this influx. Cloud computing has become a popular solution for data storage but also introduces challenges such as latency, cost, and data control.
Data management strategies need to be scalable and adaptable. Manufacturers must carefully consider how to handle data growth without sacrificing speed or efficiency. Implementing solutions that enable real-time analytics while keeping costs manageable is essential for long-term success.
Skill Gaps and Low Adoption Rates
Even with the best technology in place, a lack of skilled personnel can hinder the adoption of data-driven manufacturing. Traditional manufacturing roles often don't require advanced data analytics skills, and many workers may be unfamiliar with interpreting complex datasets. As a result, companies may face resistance to adopting new technologies or find that the available workforce isn't equipped to work with these systems.
To address this challenge, manufacturers must invest in training and development programs that upskill their workforce. Additionally, incorporating user-friendly, no-code, or low-code platforms can help lower the barrier to entry for employees who may not have a solid technical background.
High Total Cost of Ownership (TCO)
While data-driven manufacturing can lead to significant cost savings in the long run, the initial implementation costs can be high. Investing in new equipment, software, and cybersecurity measures requires capital, and the return on investment might not be immediate. Additionally, ongoing costs, such as system maintenance, cloud storage fees, and data analytics tools, can add to the overall TCO.
To manage these costs, manufacturers must take a phased approach, focusing on high-impact areas first. By starting with smaller pilot projects, companies can prove the value of data-driven manufacturing before scaling up, ensuring that each process step is affordable and effective.
Change Management
Managing change is one of the toughest challenges in adopting data-driven manufacturing, especially at the operational level. Employees on the shop floor may resist new technologies or feel overwhelmed by the shift to data-centric processes.
To ensure success, manufacturers must invest in proper training, create clear communication channels, and actively involve workers in the transition. By fostering a culture of openness and providing continuous support, organizations can make data-driven practices more accessible and efficient for everyone, from the top down to the operators.
Domain Data Mapping
Data-driven manufacturing isn’t just about technology—it’s also about translating data into meaningful insights for business decisions. Domain data mapping ensures that data professionals understand the manufacturing process deeply, aligning technical insights with business objectives.

For instance, data scientists need to know how specific metrics affect production efficiency or product quality. Manufacturers can derive relevant insights that directly support process optimization by bridging the gap between data experts and operational teams, improving strategic and operational outcomes.
Despite the hurdles, data remains an invaluable asset in manufacturing. Let’s check why.
Why Data Is a Game-Changer in Manufacturing?
Data has transformed from a supporting role into the powerhouse that fuels innovation and keeps manufacturing operations running smarter and sharper than ever. By leveraging data from machinery, workflows, and customer interactions, manufacturers unlock valuable insights that drive efficiency, quality, and agility.
Here’s how data transforms manufacturing:
- Informed Decisions, Every Time: Accurate data takes the guesswork out of decision-making, enabling manufacturers to implement strategies that yield tangible results.
- Uncovering Bottlenecks: Analytics highlight inefficiencies, helping businesses fine-tune workflows and optimize resource use.
- Cost-Quality Balance: Data reveals areas to reduce costs while maintaining or even improving product quality standards.
- Proactive Maintenance: Predictive insights anticipate equipment issues, minimizing unplanned downtime and extending machinery life.
- Real-Time Adaptability: When demand fluctuates or market conditions change, real-time data enables immediate adjustments to production schedules.
- Driving Continuous Growth: By tracking trends and monitoring performance over time, manufacturers refine operations to align with evolving goals.
- Enhancing Product Standards: Insights from production trends help detect and eliminate potential quality issues before they escalate.
With data’s value in mind, let’s look at five practical steps to implement data-driven manufacturing.
5 Steps to Use Data-Driven Manufacturing
Optimizing a supply chain is about speed and understanding where delays and inefficiencies lie. Analyzing specific factors within production processes can help manufacturers pinpoint where time is lost, uncover hidden opportunities, and set the stage for sustainable improvements.
Identify Time Bottlenecks
Lead time is often the clearest indicator of underlying issues within the supply chain. By examining areas where operations slow down, manufacturers can determine the root causes of these bottlenecks, assess their impact, and find ways to address them. The first step to efficient manufacturing is removing recurring delays and maximizing uptime.
Leverage Existing Data Sources
While additional data collection is beneficial, manufacturers already hold valuable information that can be analyzed for insights. Financial, operational, and physical data are all sources that process engineers and continuous improvement teams can use to initiate improvements. Analyzing existing data helps manufacturers build the necessary skills and infrastructure for handling more extensive data analysis in the future.
Use AI to Uncover Key Insights
Gathering data is one part of the challenge; the next step is identifying actionable insights. AI-driven analytics offer faster and more accurate interpretations than manual analysis, leading to significant improvements. Studies show that AI-enhanced analytics can reduce order-to-delivery times and dramatically boost supply chain efficiency, making it an invaluable tool for complex decision-making.
Illuminate Unknown Areas with Sensor Data
Even top manufacturing facilities have blind spots in their operations. Connected sensors help illuminate these unknowns, offering visibility into previously hard-to-monitor areas. This technology provides the data needed to improve processes and uncover new optimization opportunities.
Balance Automation with Flexibility
As manufacturers increasingly rely on data, there’s a tendency to automate wherever possible. However, full automation isn’t ideal for every scenario. For companies with unpredictable demand, maintaining some operational flexibility allows them to adapt more quickly to changes rather than being constrained by a rigid, fully automated system.
Finally, let’s dive into future strategies to keep data-driven manufacturing evolving.
Future Strategies for Data-Driven Manufacturing
As manufacturers increasingly rely on data to drive decisions, future strategies must evolve to support efficiency and innovation. Here are some key future strategies that will help overcome the challenges and shape the future of data-driven manufacturing:
Adoption of No-Code/Low-Code Tools
One emerging trend is the use of no-code and low-code platforms, which make data tools more accessible to non-technical staff. These platforms allow workers to interact with complex data systems without in-depth coding skills.

For example, a production manager could use a no-code dashboard to monitor machine performance and make real-time adjustments without involving the IT department. This ease of use accelerates adoption and lowers the skill gap, allowing manufacturers to implement data-driven solutions across the workforce.
INSIA’s no-code data operations, management and analytics platform that empowers users of all skill levels to quickly build and interact with data dashboards and analysis.. With INSIA’s tool, organizations can visualize, interpret, and leverage data insights without any programming knowledge, removing a common barrier to effective data utilization.
By democratizing access to data visualization, INSIA enables teams across all departments to make faster, insight-driven decisions. Learn more about INSIA’s - easy dashboarding here.
Unified Platforms for Seamless Integration
As manufacturers struggle with fragmented systems, the need for unified platforms becomes more critical. A unified platform allows all data from production lines, suppliers, or customer feedback to be integrated into one system. This reduces the complexity of multiple datasets and gives manufacturers a holistic view of their operations.
A centralized data platform can help manufacturers ensure more streamlined operations, reduce manual interventions, and create a more cohesive decision-making process.
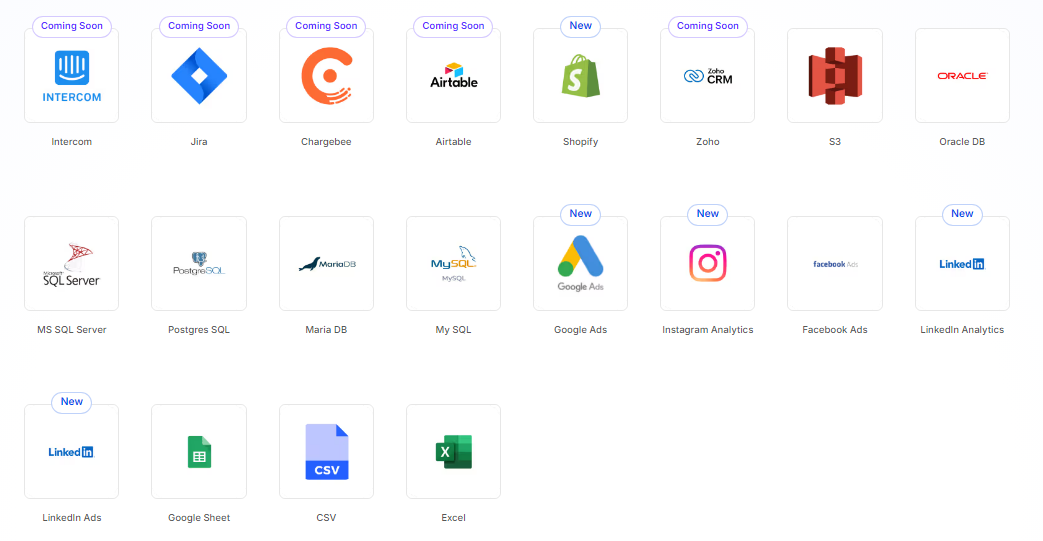
Industry-Specific Data Solutions
Moving forward, industry-specific data solutions will become essential. These solutions are tailored to address particular sectors' unique needs and challenges, such as automotive, electronics, or pharmaceuticals.
For example, in the automotive industry, a domain-specific solution might focus on optimizing supply chain logistics and ensuring just-in-time (JIT) delivery. Manufacturers benefit from tools designed specifically for their operations, enabling them to address general and industry-specific challenges more effectively.

Building a Continuous Improvement Framework
Finally, future strategies should focus on creating a continuous improvement framework based on data-driven insights. Rather than implementing static solutions, manufacturers must embrace a culture of ongoing optimization.
This means regularly analyzing performance metrics, customer feedback, and production data to identify areas for improvement. By doing so, manufacturers can consistently refine processes and stay competitive and responsive to market changes or technological advancements.
Conclusion
Data-driven manufacturing is crucial for sustainable growth but comes with challenges like managing siloed data and legacy systems. However, with the right strategies, manufacturers can overcome these barriers and unlock new opportunities.
Here's how INSIA can help with data-driven manufacturing analytics:
- Seamless Data Integrations: INSIA enables easy data connection across multiple systems, improving operational visibility and decision-making.
- Data Transformation Tools: Simplify raw data conversion into meaningful insights using intuitive platforms.
- Search-Driven Analytics: Use AI to quickly analyze data, allowing for accurate insights without needing technical skills.
- Automated Reporting: INSIA offers customizable, automated reports that save time and improve tracking.
- AI-Powered Predictions: Optimize processes with predictive AI tools to identify trends and streamline operations.
- Robust Data Governance: Ensure data security and compliance with customizable governance tools.
- Mobile-Ready Access: Get real-time insights on the go with mobile-friendly dashboards and alerts.